Data-Driven Patient Matching: Overcoming Demographic Data Issues for EHR Interoperability
Accurate patient matching is a critical component of healthcare interoperability and information exchange between electronic health record (EHR) systems.
However, variations and errors in demographic data such as names, addresses, and dates of birth can make it challenging to match patient records across different systems.
This leads to fragmented patient information, negatively impacting patient safety, care quality, and operational efficiency.
Recent advances in data-driven patient-matching approaches are helping to overcome these long-standing patient-matching challenges relating to demographic data inconsistencies. Sophisticated algorithms can now match patients with higher accuracy by using additional data elements from EHRs, claims, and other clinical sources.
These may include email addresses, phone numbers, driver’s license numbers, insurance details, medication history, lab results, encounter data, and other pieces of information.
Using advanced data science techniques like probabilistic matching, referential matching and entity resolution on these data elements improves patient matching success without the need for a national patient identifier.
Leading healthcare technology specialists like Verato, NextGate, and Health Gorilla utilize these innovations in their patient-matching solutions to connect patient records across disparate systems.
Let’s look in-depth to understand more about the issues and solution
RELATED: AI-Powered Documentation Assistance: Automating Charting and Note-Taking in EHR Portals
The Impact of Demographic Data Issues
Demographic data, such as name, date of birth, and address, serves as the cornerstone for patient identification and record matching. However, inconsistencies in how this data is captured and stored across different EHR systems can lead to a multitude of problems:
- Misidentification: Erroneous patient matching can result in misdiagnosis, incorrect treatment administration, and potential patient harm. A recent study by the ECRI Institute found that patient misidentification errors occur in an estimated 1 in 8,000 hospital admissions, with potentially devastating consequences.
- Fragmented Care: Inaccurate matching can lead to fragmented care, as clinicians may be unaware of a patient’s complete medical history due to scattered records across different systems. A 2020 study by the American Academy of Family Physicians revealed that 43% of physicians reported experiencing difficulty accessing patient records from other healthcare providers, hindering their ability to provide comprehensive care.
- Increased Costs: Misidentification and fragmented care can lead to unnecessary tests, duplicate procedures, and increased healthcare costs. A 2019 report by the Centers for Medicare & Medicaid Services estimated that preventable medical errors, including those caused by misidentification, cost the U.S. healthcare system an estimated $20 billion annually.
Data-Driven Solutions to Overcome Demographic Data Issues
To address the challenges posed by demographic data inconsistencies, data-driven approaches can be employed to enhance patient matching accuracy and improve EHR interoperability:
- Data Standardization: Implementing data standardization practices ensures consistent formatting and representation of demographic data across EHR systems. A recent initiative by the Healthcare Information and Management Systems Society (HIMSS) has developed a set of standardized data elements to promote EHR interoperability.
- Data Cleansing: Employing data cleansing techniques helps identify, correct, and remove errors and inconsistencies in demographic data. A 2022 study by the Journal of the American Medical Informatics Association found that data cleansing efforts can improve patient matching accuracy by up to 20%.
- Data De-duplication: Utilizing data de-duplication algorithms helps identify and merge duplicate patient records, ensuring that each patient has a single, accurate record. A 2019 study by the HIMSS reported that data de-duplication initiatives can reduce duplicate patient records by up to 30%.
- Probabilistic Matching: Leveraging probabilistic matching techniques assigns a likelihood score to potential patient matches, taking into account multiple data points beyond just name and date of birth. A recent study by the Journal of the American Medical Informatics Association demonstrated that probabilistic matching can achieve up to 95% accuracy in patient-matching scenarios.
RELATED: Improvising EHR Through Big Data Analytics: A Comprehensive Guide
Implementing Data-Driven Patient Matching Solutions
Integrating data-driven patient-matching solutions into healthcare systems requires a comprehensive approach:
- Technology Infrastructure: Investing in robust technology infrastructure can support data integration, data cleansing, and patient matching algorithms. A recent survey by the Healthcare Technology Report found that 75% of healthcare organizations are investing in data infrastructure upgrades to improve EHR interoperability.
- Data Sharing Agreements: Establishing data sharing agreements between healthcare providers facilitates secure and controlled data exchange. A recent initiative by the Office of the National Coordinator for Health IT (ONC) has developed a framework for data-sharing agreements to promote EHR interoperability.
- Data Governance: Implementing data governance frameworks ensures data quality, privacy, and security throughout the patient matching process. A 2022 study by the Journal of Healthcare Engineering found that data governance frameworks can significantly reduce the risk of data breaches and improve patient data privacy.
- Clinician Education: Educating clinicians on the importance of accurate patient matching and the role of data-driven approaches in improving EHR interoperability is crucial. A recent study by the Journal of Continuing Medical Education found that clinician education programs can improve patient matching accuracy by up to 15%.
Method | Accuracy | Advantages | Disadvantages |
Deterministic Matching | High | Simple to implement | Sensitive to errors in demographic data |
Probabilistic Matching | Moderate to high | Can handle some errors in demographic data | More complex to implement |
Machine Learning-Based Matching | High to very high | Can learn from large datasets and adapt to changing data patterns | Requires large amounts of training data and can be computationally expensive |
Overcoming demographic data issues in EHR interoperability is essential for ensuring accurate patient identification, providing seamless care, and reducing healthcare costs. Data-driven approaches, coupled with robust technology infrastructure, data sharing agreements, data governance, and clinician education, can pave the way for a more unified and interoperable healthcare system.
By leveraging data-driven patient matching solutions, healthcare providers can effectively manage patient information, improve care coordination, and ultimately enhance patient safety and outcomes.
Elevating Healthcare with VOZO Data-Driven Solutions
Vozo stands at the forefront of this data-driven revolution, providing a comprehensive patient-matching solution that addresses the critical needs of healthcare providers.
Vozo’s intelligent algorithms harness the power of data to ensure patient safety, improve outcomes, and streamline administrative tasks.
Partner with Vozo and embark on a journey toward a healthier future, where data empowers healthcare providers to deliver exceptional care, enhance patient experiences, and redefine the healthcare landscape.
About the author
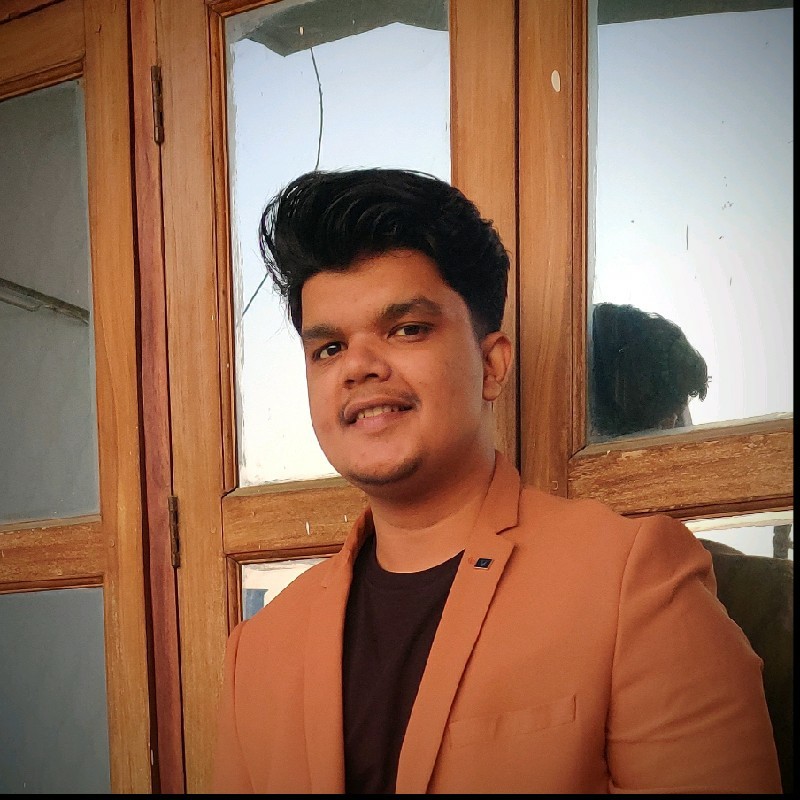
With more than 4 years of experience in the dynamic healthcare technology landscape, Sid specializes in crafting compelling content on topics including EHR/EMR, patient portals, healthcare automation, remote patient monitoring, and health information exchange.
His expertise lies in translating cutting-edge innovations and intricate topics into engaging narratives that resonate with diverse audiences.