NLP EHR Integration: The Benefits, Challenges, Solutions, and Use Cases
Natural Language Processing (NLP) in Electronic Health Records (EHR) holds great significance in transforming healthcare by leveraging the power of language understanding and analysis.
RELATED: EHR and FHIR: 5 Challenges & Solutions in Managing Acute Diseases
NLP allows computers to interpret and process human language, enabling the extraction of valuable information from unstructured clinical text in EHRs. Here are the key aspects of NLP in EHR, including its significance, challenges, potential solutions, and use cases.
Significance of NLP in EHR
1. Enhanced Data Utilization
RELATED: Is it True? EHR Workflow Prompt Can Boost Lung Cancer Screening?
- NLP enables the extraction of valuable insights from clinical narratives, progress notes, discharge summaries, and other unstructured text
- This enhances the utilization of EHR data for research, analytics, and decision-making
2. Improved Clinical Decision Support
- NLP can assist healthcare providers in identifying critical information, such as diagnoses, medications, and treatment plans, from unstructured text
- This information can then be used to enhance clinical decision support systems, aiding in more accurate and timely diagnoses and treatment recommendations
3. Population Health Management
- By analyzing large volumes of unstructured EHR data through NLP, healthcare organizations can identify patterns and trends in population health
- This helps them develop targeted interventions and preventive strategies
4. Adverse Event Detection
- NLP can be employed to detect adverse events and potential safety issues in EHRs by analyzing unstructured narratives
- Thereby contributing to patient safety and quality improvement efforts
Challenges of NLP in EHR
1. Unstructured Data
- EHRs often contain a significant amount of unstructured text
- This makes it challenging for traditional computer systems to process and extract meaningful information
2. Variability in Language
- Healthcare professionals may use different terms, abbreviations, or synonyms to describe the same medical concepts
- NLP systems must be robust enough to handle this variability
3. Contextual Understanding
- Understanding the context and intent of the language is complex
- Especially in the medical domain where ambiguity can exist in clinical narratives
4. Data Privacy and Security
- Extracting information from unstructured text raises concerns about patient privacy and data security
- This necessitates robust de-identification and anonymization techniques
Solutions to NLP Challenges in EHR
1. Advanced NLP Algorithms
Developing and employing advanced NLP algorithms like deep learning models, can improve the accuracy and effectiveness of language understanding.
2. Ontologies and Standardization
Creating standardized medical vocabularies and ontologies can help address the challenge of varied language use, making it easier for NLP systems to interpret clinical text.
3. Contextual Analysis
Implementing context-aware NLP models can enhance the understanding of language and improve accuracy in extracting medical concepts from EHRs.
4. Privacy-Preserving Techniques
Implementing privacy-preserving NLP methods, like differential privacy and secure multi-party computation, can protect patient data while still allowing useful analysis.
5. Integration with Clinical Workflows
Seamlessly integrating NLP tools into clinical workflows can enhance their usability and adoption among healthcare professionals.
6. Continuous Training and Improvement
Regularly updating and retraining NLP models using new data can improve their performance and keep them up-to-date with the latest medical terminology and language use.
NLP Practical Use Cases that Benefit Providers, Researchers, and Patients
1. Clinical Documentation Automation
- NLP can automatically extract relevant information from clinician notes and convert it into structured data, populating various sections of the EHR (e.g., diagnoses, medications, lab results)
- This automation reduces the burden on providers, streamlines documentation processes, and ensures the accuracy of recorded information
2. Clinical Decision Support
- NLP can analyze patient data, including clinical notes, past medical history, and lab results, to provide real-time decision support to healthcare professionals
- It can alert clinicians to potential drug interactions, suggest appropriate treatment plans, and offer evidence-based recommendations based on the latest medical research
3. Disease Surveillance and Outbreak Detection
- NLP can monitor and analyze EHR data to detect disease outbreaks or track the spread of infectious diseases
- It can identify clusters of symptoms and patterns indicative of emerging health issues, allowing public health authorities to take timely preventive measures
4. Adverse Event Detection
- NLP can help identify adverse events and potential patient safety issues by analyzing unstructured clinical narratives
- It can assist in monitoring medication side effects, surgical complications, or adverse reactions to treatments
5. Patient Phenotyping and Cohort Identification
- NLP can be used to identify patients with specific characteristics or conditions from large EHR datasets
- This enables researchers to create patient cohorts for clinical trials, epidemiological studies, or population health research more efficiently
6. Predictive Analytics
- By processing and understanding unstructured data in EHRs, NLP can support predictive analytics models
- These models can help predict disease progression, readmission risk, or patient outcomes, aiding in proactive and personalized patient care
7. Population Health Management
- NLP can be utilized to extract and analyze social determinants of health from unstructured data in EHRs
- This enables healthcare organizations to better understand the health needs of specific populations and design targeted interventions
8. Sentiment Analysis and Patient Feedback
- NLP can be applied to analyze patient feedback and reviews, both in written and spoken forms
- This helps providers gain insights into patient satisfaction and identify areas for improvement in their services
9. Billing and Coding
- NLP can assist in automating coding and billing processes by extracting relevant information from clinical notes and mapping it to appropriate codes for reimbursement purposes
10. Clinical Research and Evidence Synthesis
- NLP can aid researchers in systematically reviewing vast amounts of medical literature
- This helps them extract relevant information for evidence-based decision-making and research synthesis
Final Thoughts
NLP in EHR has immense potential to unlock valuable insights from unstructured clinical text, leading to improved patient care, better clinical decision-making, and more effective population health management.
Overcoming challenges through advanced algorithms, standardization, context-awareness, and privacy-preserving techniques will be crucial in maximizing the benefits of NLP in healthcare.
NLP in EHR offers a wide range of use cases that can significantly improve the efficiency and quality of healthcare delivery, support evidence-based medicine, and enhance population health management. By unlocking the value of unstructured clinical data, NLP empowers healthcare professionals and researchers with valuable insights to make informed decisions and improve patient outcomes.
Cloud-based EHR from Vozo
Time is the main barrier that keeps healthcare professionals away from their patients and the delivery of quality care to them. Vozo EHR lets you concentrate on your patients despite your busy schedules through effective implementation and integration and makes valuable time for you to grow your hospital.
- Vozo’s Cloud EHR acts as the backbone for all your hospital needs
- We tailor features to correct and speed up your workflow for providing quality care
- Whether you’re implementing new software or replacing your current EHR, we have you all covered
- Our Specialty EHR tends to the specific requirement of your hospital’s each specialty
Our customizable templates let physicians view patient records & create case notes and more from a single screen. Vozo RCM, Patient Portal, & Telehealth integrated with EHR & Healthcare Practice Management software offers greater efficiency and cost savings across the board. Our specialty-specific tools improve workflow, billing, scheduling, and documentation.
Our features include e-prescribing, security, patient portals, lab integration, cloud hosting, and more. We offer the best means of ICD-10 standards which minimizes your administrative work.
“Let’s make your beaming practice reach more on time to all with our integrated Cloud EHR software”
About the author
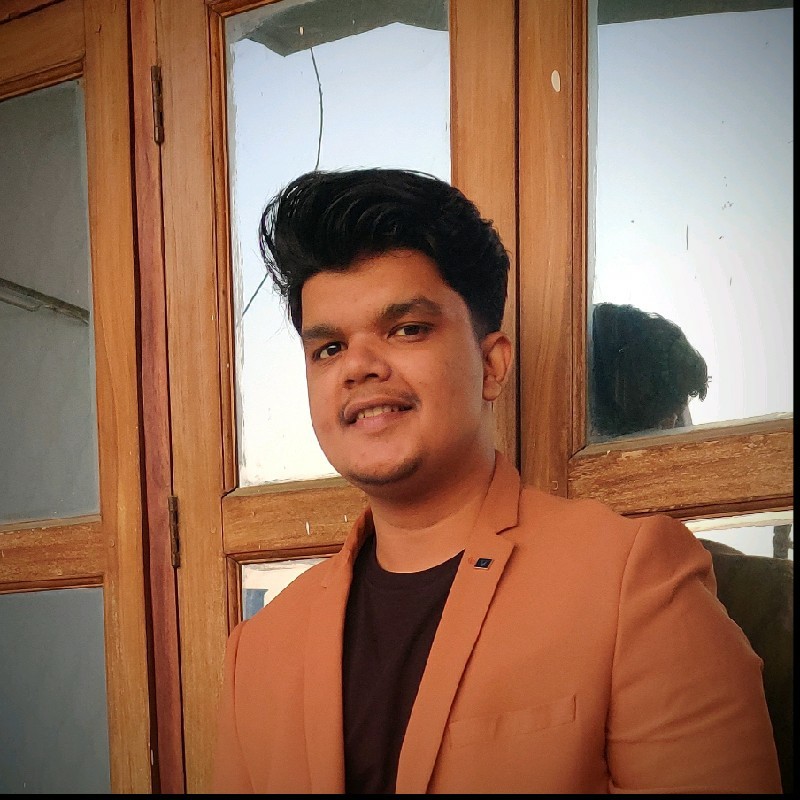
With more than 4 years of experience in the dynamic healthcare technology landscape, Sid specializes in crafting compelling content on topics including EHR/EMR, patient portals, healthcare automation, remote patient monitoring, and health information exchange.
His expertise lies in translating cutting-edge innovations and intricate topics into engaging narratives that resonate with diverse audiences.